Navigating Uncharted Waters: The latent risks of AI in Product Development
- Eva Frankenberger
- Mar 4, 2024
- 2 min read
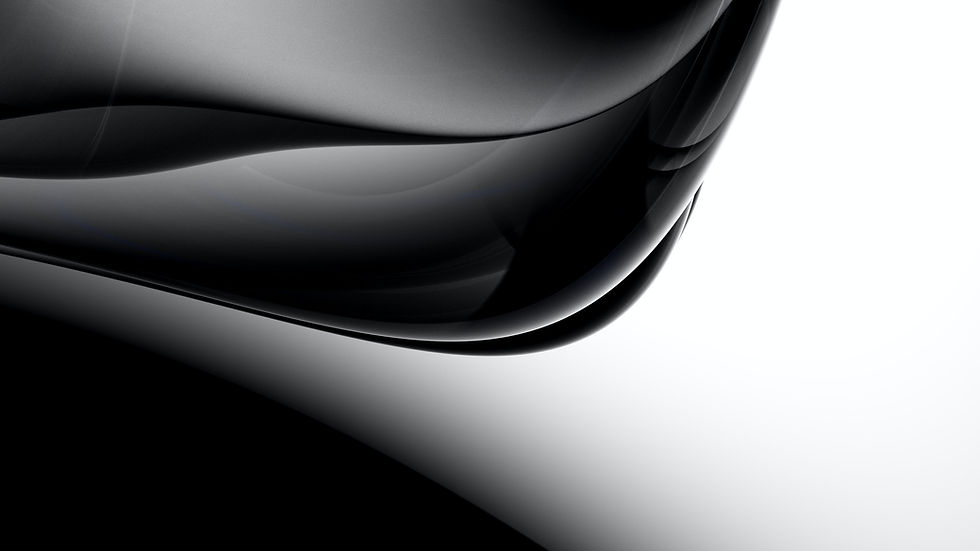
Navigating the complex landscape of Artificial Intelligence (AI) in product development or in-house AI utilization, organizations face unique cybersecurity challenges that demand a nuanced understanding and strategic approach. This article highlights the latent risks associated with AI, focusing on the aspects often overlooked yet crucial for CROs, CTOs, CEOs, and particularly CISOs, to ensure the integrity and security of AI-powered products.
The complexity of AI systems presents a significant challenge for cybersecurity teams. The intricate data patterns and algorithms that drive AI operations are not always well-understood, creating a gap in effective oversight and defense mechanisms against potential security breaches.
Adversarial attacks pose a notable risk, where malicious actors manipulate input data, causing AI to produce incorrect outcomes. Such vulnerabilities, unless properly addressed, can significantly impact the reliability of AI-driven decisions. Similarly, data poisoning, where training data is intentionally skewed, can compromise the integrity and bias outcomes of AI models, often remaining undetected until it affects decision-making processes. The "black box" nature of many AI models complicates the task of understanding the rationale behind AI decisions, challenging incident response efforts and the ability to audit AI systems for security and fairness. Moreover, the reliance on third-party datasets and pre-trained models introduces risks of integrating vulnerabilities or biases into AI solutions, potentially leading to security breaches or ethical dilemmas. To address these challenges, a paradigm shift in AI security is required, emphasizing the need for closer collaboration between AI developers and cybersecurity teams. Bridging the knowledge gap through targeted education and interdisciplinary training is essential. Implementing a lifecycle approach to AI security, from design and development through deployment and monitoring, can enhance system resilience.
For CISO teams, developing expertise in AI-specific security concerns is vital. This includes a deep understanding of AI model behavior, adversarial attack vectors, and data protection strategies in AI contexts. Familiarity with AI interpretability and explainability tools, such as SHAP, LIME, and Grad-CAM, is crucial for analyzing and understanding model decisions, alongside a solid foundation in AI and ML principles and data science skills.
Recognizing and addressing the unique cybersecurity challenges posed by AI is paramount for organizations to safeguard their AI-driven innovations. Enhancing the synergy between AI development and cybersecurity practices will not only protect against emerging threats but also secure a competitive edge in the AI-centric landscape. The journey towards AI resilience, marked by informed leadership and strategic collaboration, is complex yet essential for navigating the uncharted waters of AI in product development with confidence.
Comments